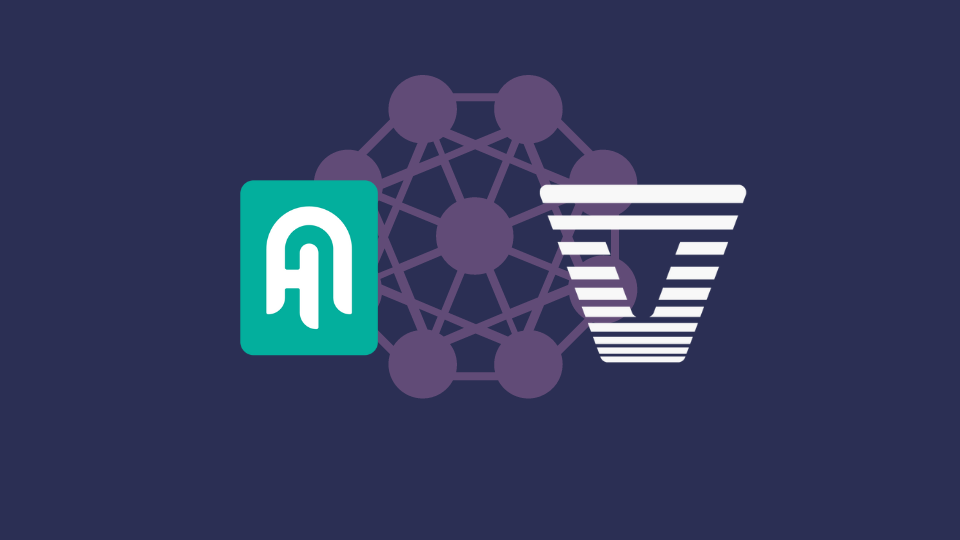
Use Gradient Models with Haystack Pipelines
Introducing the new Haystack Integration, allowing you to easily fine-tune and deploy models on the Gradient platform which you can use in Haystack pipelines
December 11, 2023One of the more cumbersome aspects of creating LLM applications is model management. Especially in cases where we need to fine-tune, host, and scale the models ourselves. In this case, having options at hand can be great. Today, we’ve expanded the Haystack 2.0 ecosystem with a new integration that can help you with just that
Gradient is an LLM development platform that offers web APIs for fine-tuning, embeddings, and inference on state-of-the-art open-source models. In this article, let’s take a look at the new Gradient integration for Haystack, and how you can use it in your retrieval-augmented generative pipelines.
You can find an example Colab here, which uses embedding and generative models from Gradient for a RAG pipeline on Notion pages.
What the Gradient Integration Provides
The Gradient Integration for Haystack comes with three new components for Haystack pipelines:
- The
GradientDocumentEmbedder
: You can use this component to create embeddings of documents. - The
GradientTextEmbedder
: You can use this component to create embeddings for text snippets such as queries. - The
GradientGenerator
: You can use this component to generate responses using LLMs.
How to use the Gradient Integration
The Gradient platform provides an embeddings endpoint (at the time of writing, it supports bge-large) and the fine-tuning and deployment of LLMs such as Llama-2, Bloom, and more (with more planned).
To fine-tune and use models via Gradient, the first step is to create a workspace.
Once you have a workspace, you will be able to select a base model and start a fine-tuning job.
Using the GradientGenerator
You can use the GradientGenerator
either with any of the available
base models that Gradient provides, or with a model that you have fine-tuned on the platform.
For example, to use the llama2-7b-chat
model:
generator = GradientGenerator(access_token='YOUR_GRADIENT_ACCESS_TOKEN',
workspace_id="YOUR_WORKSPACE_ID",
base_model_slug="llama2-7b-chat",
max_generated_token_count=350)
Or, to use a model that you’ve fine-tuned on the Gradient platform, provide your model_adapter_id
generator = GradientGenerator(access_token='YOUR_GRADIENT_ACCESS_TOKEN',
workspace_id="YOUR_WORKSPACE_ID",
model_adapter_id="your_finetuned_model_adapter_id",
max_generated_token_count=350)
Building a RAG pipeline
In this article, I’ve provided an example
Colab that does question-answering on your private Notion pages, using the NotionExporter
integration. We use the GradientDocumentEmbedder
component to create embeddings of our notion pages and index them into an InMemoryDocumentStore
. As for the RAG pipeline, you can use the GradientTextEmbedder
and GradientGenerator
to:
- Embed the user query to retrieve the most relevant documents from our Notion pages
- Generate a response using our own fine-tuned LLM from Gradient:
from haystack.components.retrievers.in_memory import InMemoryEmbeddingRetriever
from haystack.components.builders import PromptBuilder
from haystack_integrations.components.embedders.gradient import GradientTextEmbedder
from haystack_integrations.components.generators.gradient import GradientGenerator
prompt = """ Answer the query, based on the
content in the documents.
Documents:
{% for doc in documents %}
{{doc.content}}
{% endfor %}
Query: {{query}}
"""
text_embedder = GradientTextEmbedder(access_token='YOUR_GRADIENT_ACCESS_TOKEN',
workspace_id="YOUR_WORKSPACE_ID",)
retriever = InMemoryEmbeddingRetriever(document_store=document_store)
prompt_builder = PromptBuilder(template=prompt)
generator = GradientGenerator(access_token='YOUR_GRADIENT_ACCESS_TOKEN',
workspace_id="YOUR_WORKSPACE_ID",
model_adapter_id="your_finetuned_model_adapter_id",
max_generated_token_count=350)
rag_pipeline = Pipeline()
rag_pipeline.add_component(instance=text_embedder, name="text_embedder")
rag_pipeline.add_component(instance=retriever, name="retriever")
rag_pipeline.add_component(instance=prompt_builder, name="prompt_builder")
rag_pipeline.add_component(instance=generator, name="generator")
rag_pipeline.connect("text_embedder", "retriever")
rag_pipeline.connect("retriever.documents", "prompt_builder.documents")
rag_pipeline.connect("prompt_builder", "generator")
To run this pipeline:
question = "What are the steps for creating a custom component?"
rag_pipeline.run(data={"text_embedder":{"text": question},
"prompt_builder":{"query": question}})